The center for machine learning is providing support to several lines of business (LOB) at an enterprise level. Some of these LOBs support critical systems that require a very high level of availability; that level of service availability brings its own challenges. The key challenges include implementing (more) relevant service level indicators and objective metrics for all tenants of the platform, standardizing approaches to resolving incidents, and minimizing the downstream impact of incidents. Without addressing these issues the potential negative impacts are a degradation of service quality, inconsistent reporting and a drop in productivity. All impacts the team looked to avoid. Proper documentation of critical systems is especially problematic knowing that if a system goes down there is potential for a regulatory process to not run and key information to not reach the appropriate parties in a timely manner. These are all challenges for which we provided support, suggested improvements and action plans to mitigate.
Revolutionizing Operations: A Triumph on the Platform of a Major Financial Institution
The portion of the large financial institution that we interfaced with was the center for machine learning. Ippon provided the center for machine learning with a team in a box composed of several site reliability engineers (SRE). SRE engineers have a mixed toolbag comprised of software and devOps skills, there primary focus is to try drive high availability, reliability and scalability. These SRE engineers took up the challenge of overseeing the adoption of SLI/SLO metrics for users of the platform, standing up dashboards to properly reflect the aforementioned metrics, redefining the incident support model and gathering data about all critical services on the platform. As a result of the SREs work, the center for machine learning at the large financial institution has better metrics, tracking and reporting for incidents.
The Challenge
The Impact
Ippon provided the machine learning hub of a major financial institution with a "team in a box" comprising multiple SRE engineers. These SRE engineers took up the challenge of overseeing the adoption of SLI/SLO metrics for users of the platform, standing up dashboards to properly reflect the aforementioned metrics, redefining the incident support model and gathering data about all critical services on the platform. This process also included some knowledge sharing to help those unfamiliar with SLI/SLO concepts to understand what they are and the benefits they would bring to the platform.
Once a MVP was demoed and approved, engineers met with team leads, senior engineers and POs to determine what data was relevant and critical to be put in New Relic dashboards. Only key SLIs for all services were included so that senior leadership could understand the health of the platform at a glance. Metrics were collected from New Relic agents, cloudTrail, Snowflake and serviceNow. Redefining the support model included determining what was the scope of support. Ultimately, it was decided that support included dedicated slack support channels, email notifications, pagerduty alerts, proper ticketing, how to handle escalations, defining areas of responsibility and writing post mortems. All of these aspects had to be defined in a way that covered a majority of issues faced by the platform but also had enough flexibility to allow engineers to pivot as needed when triaging incidents.
The Delivery
Discovery workstream… (Discovery was done ad-hoc)
Product workstream… (Product work wasn’t within our scope)
Team efficiency/coaching workstream…
The approach used during this engagement was to work closely with the teams affected by the standards and changes we strove to implement.
The actions taken to drive team efficacy were:
- Team members attending our teams stand ups
- All stakeholders at planning and refinement sessions
- Raising dependencies on other teams boards
- KT sessions
- Retrospectives
- Call out blockers early
The result
As a result of the SRE engineers work within the center for machine learning, the support model was reworked to incorporate more LOBS on the platform. As a result of the New Relic dashboards running in production, incident management has higher visibility. Stakeholders can track the SLOs for individual entities and use that to influence prioritization for platform improvements.
Our latest success stories
Stock recommendation company sees accelerated data analytics with a modernized cloud platform
Read more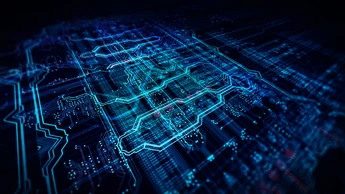
Budget-friendly Acceleration, Course Correction and Agile Coaching when a Data Lake pilot hits turbulence
Top 10 private equity firm uses a data lake transformation to also pilot agile development. Their first attempt loses steam, but Ippon takes a different approach.
Read more
Debt Financing Fintech
Accelerating a Fintech’s Data Migration to Power Data Insights for Making Impactful Business Decisions
A debt financing fintech that offers a single point-of-sale platform with a wide range of pay-over-time products and services which allow merchants to make offers to all customer types with varying levels of credit. The company credits their ability to deliver such a positive experience for merchants and customers due to their world-class support, advanced technology, and analytics that power their product. As growth continues to increase, it is vital for their company to ensure they are able to track and report on key metrics supporting business decisions.
